Abstract
Credit card companies must be capable of detecting credit card fraud transactions in order to detect fraudulent transactions of products that the customer did not buy. This has driven a lot of financial institutions to put strict actions on their customers’ using credit cards. So, Data Science and machine learning are now assisting the financial institutions with more powerful and robust machine leaning models which can help the admins identify the fraudulent transactions involved with the user’s credit card.
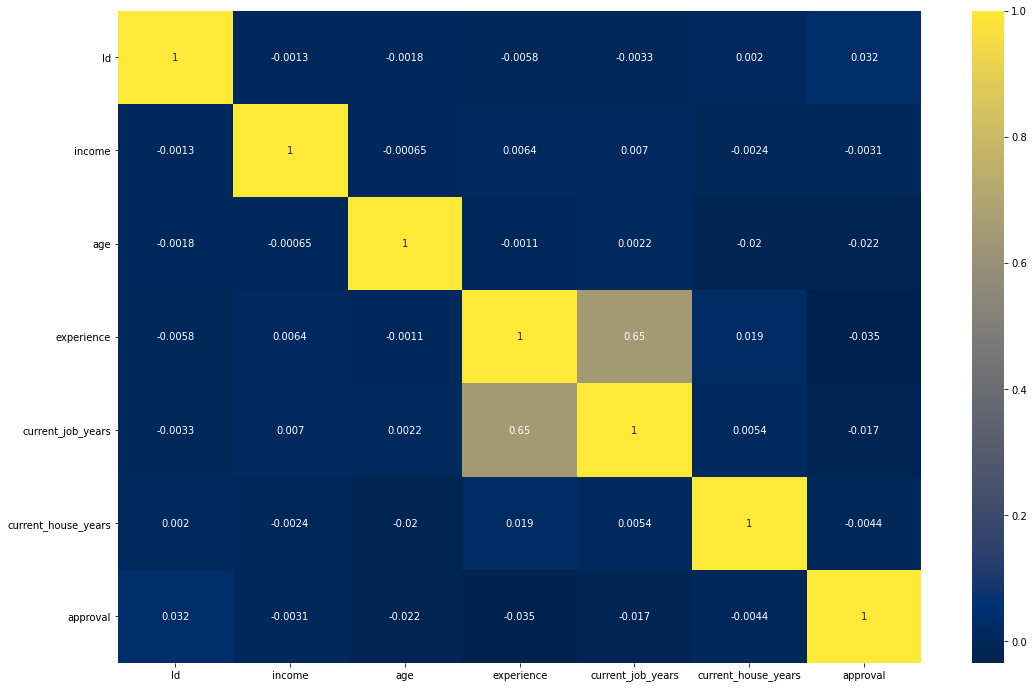
Algorithm Description
K Nearest Neighbour: KNN or K Nearest neighbours is a basic yet an efficient algorithm which is being used in most of the Machine learning application. Since it is a non-parametric i.e. This algorithm doesn’t make any underlying assumption like other algorithms do, such as having specify distribution of data to work with. So, this makes it very easy and understandable to all the users who are using it. The Technique KNN applies in predicting on new data is where it finds the nearest neighbours for the given point and takes a majority voting, whichever class is resided near to the new point, it will be considered as the new class for the new data point.
Decision Tree: This algorithm itself has the answer and explanation around it i.e., Making the decisions by splitting the question in tree like structure. Decision trees are the most widely used algorithm along with Random Forest in any machine learning project, as we have discussed above decision tree is also a non-parametric algorithm which makes it easier to understand and implement due to its capability of handling any kind of data, such as Decision tree can be applied directly on data which is not normalized/standardized, since the output/target class is predicted by taking decision from root node to leaf node, there is no need of making the data normalized/standardized. Decision tree is used in application such as Operational research, specifically in any decision analysis or to help identify a strategy to reach a particular goal.
How to Execute?
Make sure you have checked the add to path tick boxes while installing python, anaconda.
Refer to this link, if you are just starting and want to know how to install anaconda.
If you already have anaconda and want to check on how to create anaconda environment, refer to this article set up jupyter notebook. You can skip the article if you have knowledge of installing anaconda, setting up environment and installing requirements.txt
- Install the prerequisites/software’s required to execute the code from reading the above blog which is provided in the link above.
- Press windows key and type in anaconda prompt a terminal opens up.
- Before executing the code, we need to create a specific environment which allows us to install the required libraries necessary for our project.
- Type conda create -name “env_name”, e.g.: conda create -name project_1
- Type conda activate “env_name, e.g.: conda activate project_1
- Go to the directory where your requirement.txt file is present.
- cd <>. E.g., If my file is in d drive, then
- d:
7.cd d:\License-Plate-Recognition–main #CHANGE PATH AS PER YOUR PROJECT, THIS IS JUST AN EXAMPLE
8. If your project is in c drive, you can ignore step 5 and go with step 6
9. g., cd C:\Users\Hi\License-Plate-Recognition-main
10. CHANGE PATH AS PER YOUR PROJECT, THIS IS JUST AN EXAMPLE
11. Run pip install -r requirements.txt or conda install requirements.txt (Requirements.txt is a text file consisting of all the necessary libraries required for executing this python file. If it gives any error while installing libraries, you might need to install them individually.)
12. To run .py file make sure you are in the anaconda terminal with the anaconda path being set as your executable file/folder is being saved. Then type python main.pyin the terminal, before running open the main.py and make sure to change the path of the dataset.
13. If you would like to run .ipynb file, Please follow the link to setup and open jupyter notebook, You will be redirected to the local server there you can select which ever .ipynb file you’d like to run and click on it and execute each cell one by one by pressing shift+enter.
Please follow the above links on how to install and set up anaconda environment to execute files.
Data Description
The dataset was downloaded from a private data repository which might not be available now. The dataset is a .csv file splitted into train and test dataset. The train training dataset consists of around more than 2 lakh data entries of individuals from around the country. The test data consists of around 28000 data entries on individuals without the target class, which we need to predict by training a model. There are 13 columns associated with training file and 12 columns with test file. Some of the important columns which had a huge impact on the credit card approval estimation are, profession, current_house_years, house_owner, car_owner and income.
Final Results
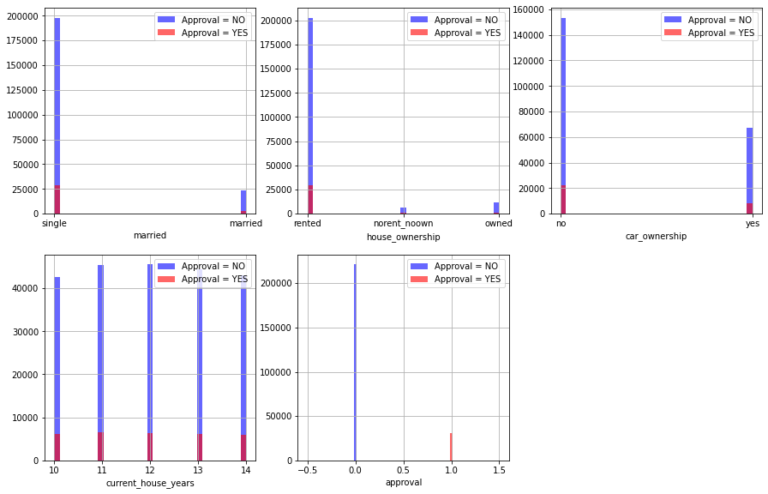
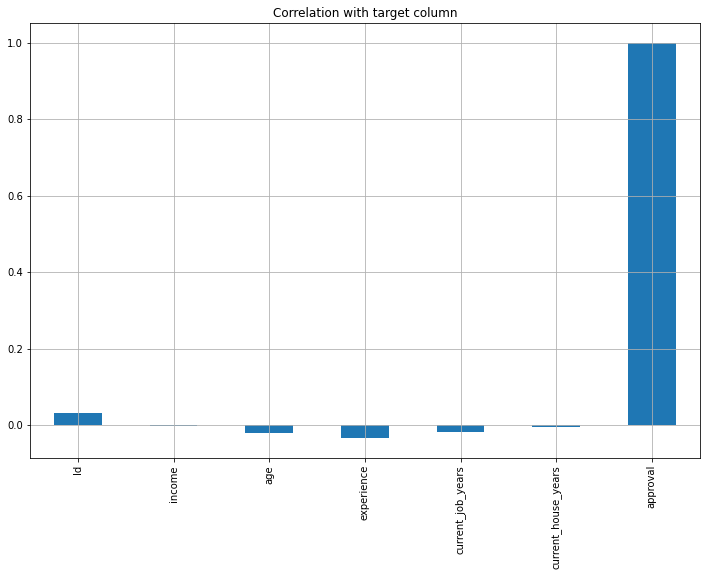
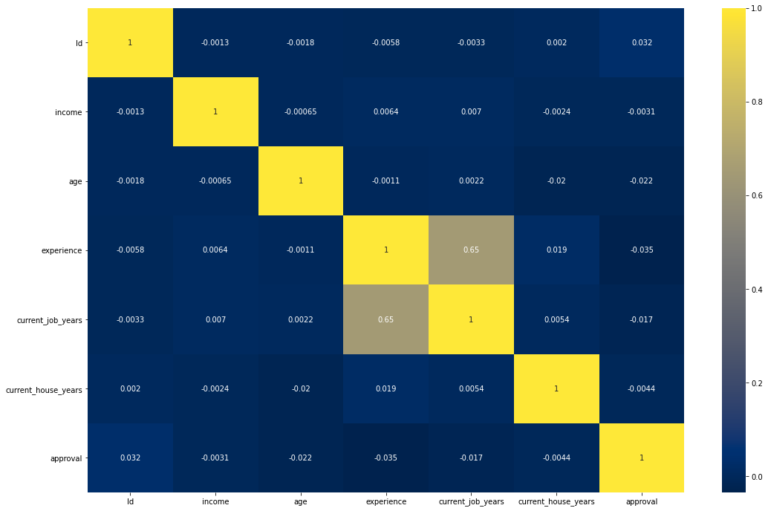
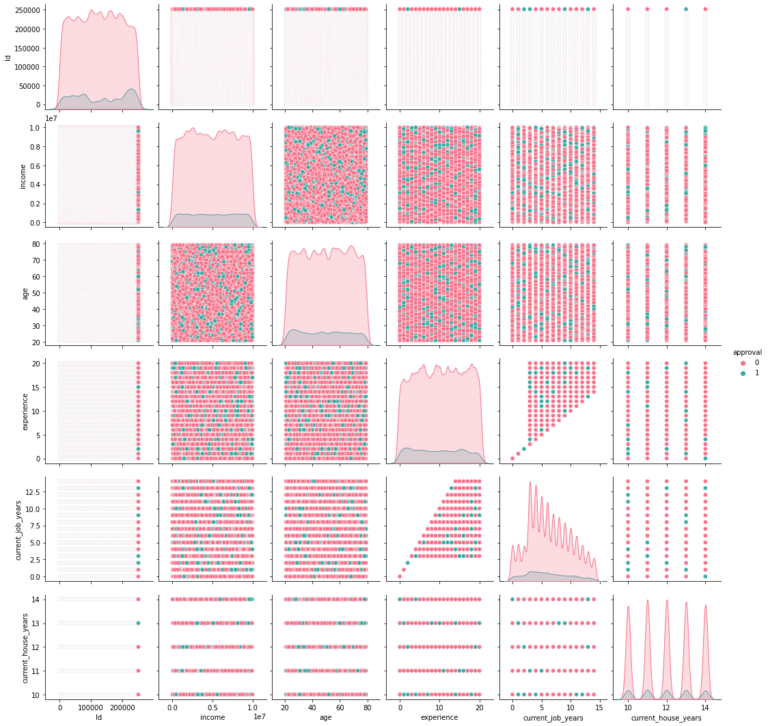
Evaluation Metric
Evaluation metrics are considered as one of the most important steps in any machine learning and deep learning projects, where it will allow us to evaluate how good our model is performing on the new data or on unseen data. There are a lot of evaluation metrics such as confusion matrix, roc_auc_curve, f1_score, recall, precision and each of which work for specific problem we deal. So, for our project we have gone with confusion matrix the OG of every evaluation matric, where using it, we have calculated the accuracy and other metric, which has given a conclusion that the model is performing very well on new data.
Confusion matrix:
Issues you may face while executing the code
- We might face an issue while installing specific libraries, in this case, you might need to install the libraires manually. Example: pip install “module_name/library” i.e., pip install pandas
- Make sure you have the latest or specific version of python, since sometimes it might cause version mismatch.
- Adding path to environment variables in order to run python files and anaconda environment in code editor, specifically in any code editor.
- Make sure to change the path in the codewhere your dataset/model is saved.
Refer to the Below links to get more details on installing python and anaconda and how to configure it.
https://techieyantechnologies.com/2022/07/how-to-install-anaconda/
Note:
All the required data has been provided over here. Please feel free to contact me for model weights and if you face any issues in executing the credit card approval detection code.
Click Here For The Source Code And Associated Files.
https://www.linkedin.com/in/abhinay-lingala-5a3ab7205/
Yes, you now have more knowledge than yesterday, Keep Going.