Abstract
Text Summarizer is an NLP Based Program where, the models try to summarize the sentence or paragraph given to it as an input. The flow goes on like this, such as, each function pre-processes the sentence in each step, removing stop words and stemming the words to remove any redundant words, the we calculate the term frequency matrix (word count), constructing a frequency matrix and giving a score to each sentence and finding out the average of each sentence and finally we generate a summary for the entire sentence

Project Methodology
As we know how text summarization helps understand a given paragraph or a long sentence easily and precisely, using natural language processing we have built a simple text summary tool which helps us to summarize any article or text we give. To make it happen there are few functions which we have followed such as sentence tokenizer, wordcount and tf_idf_matrix which is discussed briefly below. The main flow of this project goes by tokenizing each sentence, calculating the score of each sentence and generating a simple summary based on those scores.
TF-IDF – Term frequency inverse document frequency represents how important the word in a series or a corpus in a text. It also defines how proportional number of times the particular word appears in a corresponding text.
References:
How to Execute?
Make sure you have checked the add to path tick boxes while installing python, anaconda.
Refer to this link, if you are just starting and want to know how to install anaconda.
If you already have anaconda and want to check on how to create anaconda environment, refer to this article set up jupyter notebook. You can skip the article if you have knowledge of installing anaconda, setting up environment and installing requirements.txt
- Install the prerequisites/software’s required to execute the code from reading the above blog which is provided in the link above.
- Press windows key and type in anaconda prompt a terminal opens up.
- Before executing the code, we need to create a specific environment which allows us to install the required libraries necessary for our Text Summarizer project.
- Type conda create -name “env_name”, e.g.: conda create -name project_1
- Type conda activate “env_name, e.g.: conda activate project_1
- Go to the directory where your requirement.txt file is present.
- cd <>. E.g., If my file is in d drive, then
- d:
7.cd d:\License-Plate-Recognition–main #CHANGE PATH AS PER YOUR PROJECT, THIS IS JUST AN EXAMPLE
8. If your project is in c drive, you can ignore step 5 and go with step 6
9. g., cd C:\Users\Hi\License-Plate-Recognition-main
10. CHANGE PATH AS PER YOUR PROJECT, THIS IS JUST AN EXAMPLE
11. Run pip install -r requirements.txt or conda install requirements.txt (Requirements.txt is a text file consisting of all the necessary libraries required for executing this python file. If it gives any error while installing libraries, you might need to install them individually.)
12. To run .py file make sure you are in the anaconda terminal with the anaconda path being set as your executable file/folder is being saved. Then type python main.pyin the terminal, before running open the main.py and make sure to change the path of the dataset.
13. If you would like to run .ipynb file, Please follow the link to setup and open jupyter notebook, You will be redirected to the local server there you can select which ever .ipynb file you’d like to run and click on it and execute each cell one by one by pressing shift+enter.
Please follow the above links on how to install and set up anaconda environment to execute files.
Note: There are 4 different files each seeves different purpose such as,
- Preprocess.ipynb consists of all the data cleaning steps, which are necessary to build a clean and efficient model.
- main.ipynb consist of major steps and exploratory data analysis which allow us to understand more about the data and behavior of it.
- Variable_Selction.ipynb consists of data reduction/dimensionality reduction techniques such as Sequential feature selector method to reduce the dimensions in the data and compare the model scores before and after dimensionality reduction.
- Combined_main_var.ipynb consists of combination of main.ipynb and variable_selection.ipynb to make it more clear and understable for the audience.
Please follow the above sequence if you would like to execute and the files require good system requirements to run.
Make sure to change the path of the dataset in the code
Data Description
Specifically, we did not use any kind of dataset for this Text Summarizer project, since we are not using any external models/algorithms which requires some kind of dataset. The project is build using simple functions and built-in libraries.
Final Results
- Sample text summary-1

2. Sample text summary -2
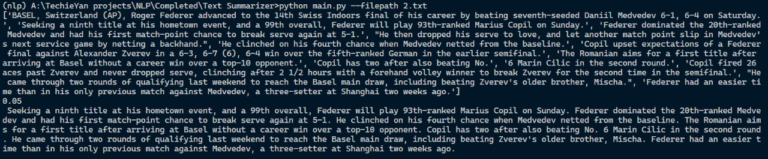
Issues you may face while executing the code
- We might face an issue while installing specific libraries, in this case, you might need to install the libraires manually. Example: pip install “module_name/library” i.e., pip install pandas
- Make sure you have the latest or specific version of python, since sometimes it might cause version mismatch.
- Adding path to environment variables in order to run python files and anaconda environment in code editor, specifically in any code editor.
- Make sure to change the paths in the code accordingly where your dataset/model is saved.
Refer to the Below links to get more details on installing python and anaconda and how to configure it.
http://techieyantechnologies.com/2022/07/how-to-install-anaconda/
Note:
All the required data has been provided over here. Please feel free to contact me for model weights and if you face any issues in executing the text summarizer.
Click Here For The Source Code And Associated Files.
https://www.linkedin.com/in/abhinay-lingala-5a3ab7205/
Yes, you now have more knowledge than yesterday, Keep Going.