Contact tracing of covid affected person using DBSCAN Clustering methodology
Abstract
Covid 19 just broke the back of the world’s economy, disrupted the normal lives of people but this is how it is. We can just adjust to it and move ahead by staying cautious. Here we created our contact tracing of covid affected person own dataset based on location coordinates as we didn’t find an appropriate dataset, then based on location coordinates we can classify them into clusters if they are within 6 feet of each other(social distancing distance) and even if one person in the cluster is covid infected or found to be covid infected, we treat all others in the same cluster as potential infections and send them message that please go for quarantine and get yourself checked.

Code Description & Execution
Algorithm Description
The task which we would be performing will be clustering i.e. grouping up of objects based on some similar properties and here the property we would be checking is that if the objects are within 6 feet of each other. If yes, they will be part of the cluster, else no.
Density-based Clustering:
In this algorithm, the clusters are formed based on the density of objects/points in the region. DBSCAN algorithm requires 2 parameters:
- eps: It defines the area around a point to consider it as a neighbor. If the distance between 2 points is less than or equal to the “eps” provided then they will be considered as neighbors.
- MinPts: It is the minimum number of points to be present to be considered as a cluster.
Reference:
How to Execute?
So, before execution we have some pre-requisites that we need to download or install i.e., anaconda environment, python and a code editor.
Anaconda: Anaconda is like a package of libraries and offers a great deal of information which allows a data engineer to create multiple environments and install required libraries easy and neat.
Refer to this link, if you are just starting and want to know how to install anaconda.
If you already have anaconda and want to check on how to create anaconda environment, refer to this article set up jupyter notebook. You can skip the article if you have knowledge of installing anaconda, setting up environment and installing requirements.txt
1. Install necessary libraries from requirements.txt file provided.

2. Go to the directory where your requirement.txt file is present.
CD<>. E.g, If my file is in d drive, then
- CD D:
- CD D:\License-Plate-Recognition-main #CHANGE PATH AS PER YOUR PROJECT, THIS IS JUST AN EXAMPLE
If your project is in c drive, you can ignore step 1 and go with step 2.
Eg. cd C:\Users\Hi\License-Plate-Recognition-main #CHANGE PATH AS PER YOUR PROJECT, THIS IS JUST AN EXAMPLE

3. Run command pip install -r requirements.txt or conda install requirements.txt
(Requirements.txt is a text file consisting of all the necessary libraries required for executing this python file. If it gives any error while installing libraries, you might need to install them individually.)
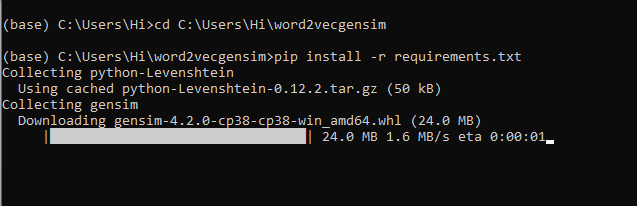
All the necessary files will get downloaded. To run the code, open anaconda prompt. Go to virtual environment if created or operate from the base itself and start jupyter notebook, open folder where your code is present.


Open “contact_tracing.ipynb” to get the results.
Data Description
No dataset with GPS locations were found. I used a mock dataset and code created by the author of this article to get the results.
Image of mock dataset

The contact tracing of covid affected person dataset contains location information (latitude and longitude) of every person along with the timestamp when they were present at that location.
https://towardsdatascience.com/contact-tracing-using-less-than-30-lines-of-python-code-6c5175f5385f
Results
1. Before clustering

2. After clustering:
Clusters formed based on the eps(minimum distance) parameter given in the code.



Issues Faced
- Ensure you have all libraries installed.
- Give correct paths wherever necessary.
- Install libraries if you get some error when installing libraries through requirements.txt
Click Here To Download This Code And Associated File.